By the end of this tutorial, you will be able to write a Named Entity Recognition pipeline using SpaCy: it will detect company acquisitions from news headlines.
Demo:

Learn on practice how to use named entity recognition to mine insights from news in real-time. We will write a Python script to analyze news articles' headlines to learn about all the latest company acquisitions.
In this tutorial, we will:
- build a data pipeline to fetch real-time news headlines
- apply pre-trained named entity recognition models provided by Spacy to identify companies that are acquired
- build an engine that tracks all companies acquisitions
Intro. NLP & Named Entity Recognition
Natural Language Processing (NLP) is a set of techniques that helps analyze human-generated text.
Examples of applying NLP to real-world business problems:
- chatbots
- text summarization
- recommendation engines
- text autogeneration
- "trading on news"
- speech recognition
We try to solve a problem in this article: find all company acquisitions from news articles published online.
Why Named Entity Recognition?
Let's compare these two articles:
"Apple is planning to acquire a news API provider in the near future"
"Apple for breakfast: pros and cons"
Both articles mention "Apple"; however, the first one talks about the company, while the second one is about the fruit.

Without named entity recognition, we are not able to even qualify the content.
There are not just organizations (ORG) that the NER can identify. Spacy - Python NLP package that we're going to use - has a pre-built NER pipeline that includes:
- DATE - absolute or relative dates or periods
- PERSON - People, including fictional
- GPE - Countries, cities, states
- LOC - Non-GPE locations, mountain ranges, bodies of water
- MONEY - Monetary values, including unit
- TIME - Times smaller than a day
- PRODUCT - Objects, vehicles, foods, etc. (not services)
- CARDINAL - Numerals that do not fall under another type
- ORDINAL - "first", "second", etc.
- QUANTITY - Measurements, as of weight or distance
- EVENT - Named hurricanes, battles, wars, sports events, etc.
- FAC - Buildings, airports, highways, bridges, etc.
- LANGUAGE - Any named language
- LAW - Named documents made into laws.
- NORP - Nationalities or religious or political groups
- PERCENT - Percentage, including "%"
- WORK_OF_ART - Titles of books, songs, etc.
For example, take a look at the article below after it's processed by the Spacy pipeline:
"On the 15th of September, Tim Cook announced that Apple wants to acquire abC Group from New York for 1 billion dollars"
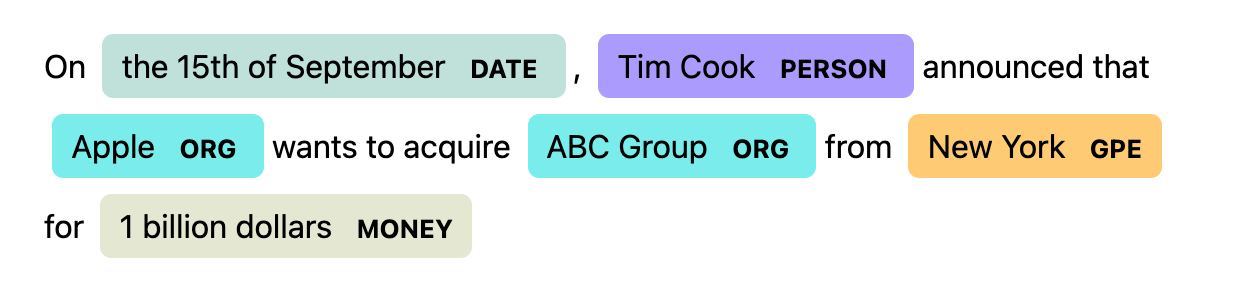
Part I. Named Entity Recognition using Spacy
For now, let's use a pre-defined list of news article headlines to test named entity recognition in Spacy.
In the case of one company that acquires another one, it is fair to assume that there should be at least two ORG tags per headline: at least one for the acquire and at least one for the acquirer.
To install Spacy, run in your console:
pip install spacy
python -m spacy download en_core_web_lg
In your Python interpreter, load the package and pre-trained model:
First, let's run a script to see what entity types were recognized in each headline using the Spacy NER pipeline.
The very first example is the most obvious: one company acquires another one. Spacy NER identified both companies correctly.

The third article headline talks about an organization and a person.

All headlines talk about some acquisition; however, not all talk about one company acquiring another one.
1. Function to filter only the articles with two ORG entities (and "acquire" in-between)
Also, let's make it a generic function that we can apply to no matter which article.
The function below does two things:
- detects if a lemma "acquire" is present in the sentence. We want to use lemma - a canonical form of a word - to make sure we cover cases as "acquired", "acquires", etc.
- check that at least 2 ORG are found
Limitations:
- can be applied only to one sentence (not on a text)
- accuracy depends on a NER that you use
Analyzing the dependency using Spacy
Now that we know that a headline is an excellent candidate for an acquisition example, we want to ensure that one company is acquiring another one. For this, we will use dependency parsing with Spacy.
I'm not going to go much into the detail as it is outside of the scope of this article.
Using display, we can visualize the dependency tree.


2. Function to detect a company(ies) to be bought
The company that is bought should be:
- of entity type ORG
- with "head" lemma being "acquire"
- independency of "attr" or "dobj"
After identifying the token, we:
- take its noun chunk
- check for "siblings": other companies that can be bought as well
3. Function to detect an acquiring company(ies)
4. Combine functions and test
Part II. Getting Real-Time Headlines with News API
I'll provide a quick example of how you could get the latest articles that contain lemma "acquire" from 2 sources: prnewswire and businesswire.
We'll use NewsCatcher News API Python SDK for that.
To install News API SDK, run in your console:
pip install newscatcherapi
You can grab your API key here.
We'll make a call to find articles that:
- contain lemma "acquire" in title
- published by prnewswire.com or businesswire.com
- published in the past 24 hours
- are written in English
Finally, we print out the results.
I leave you here so that you can experiment with actual data yourself.
A few notes on my Spacy NER accuracy with "real world" data
- Low accuracy overall, even with a large model
- You'd need to fine-tune your model if you want to use it in production
- Overall, there's no open-source high accuracy NER model that you can use out-of-a-box